PEOPLE AND CULTURE
BLOG
AI in safety, health and wellbeing – the ethics, challenges and pitfalls
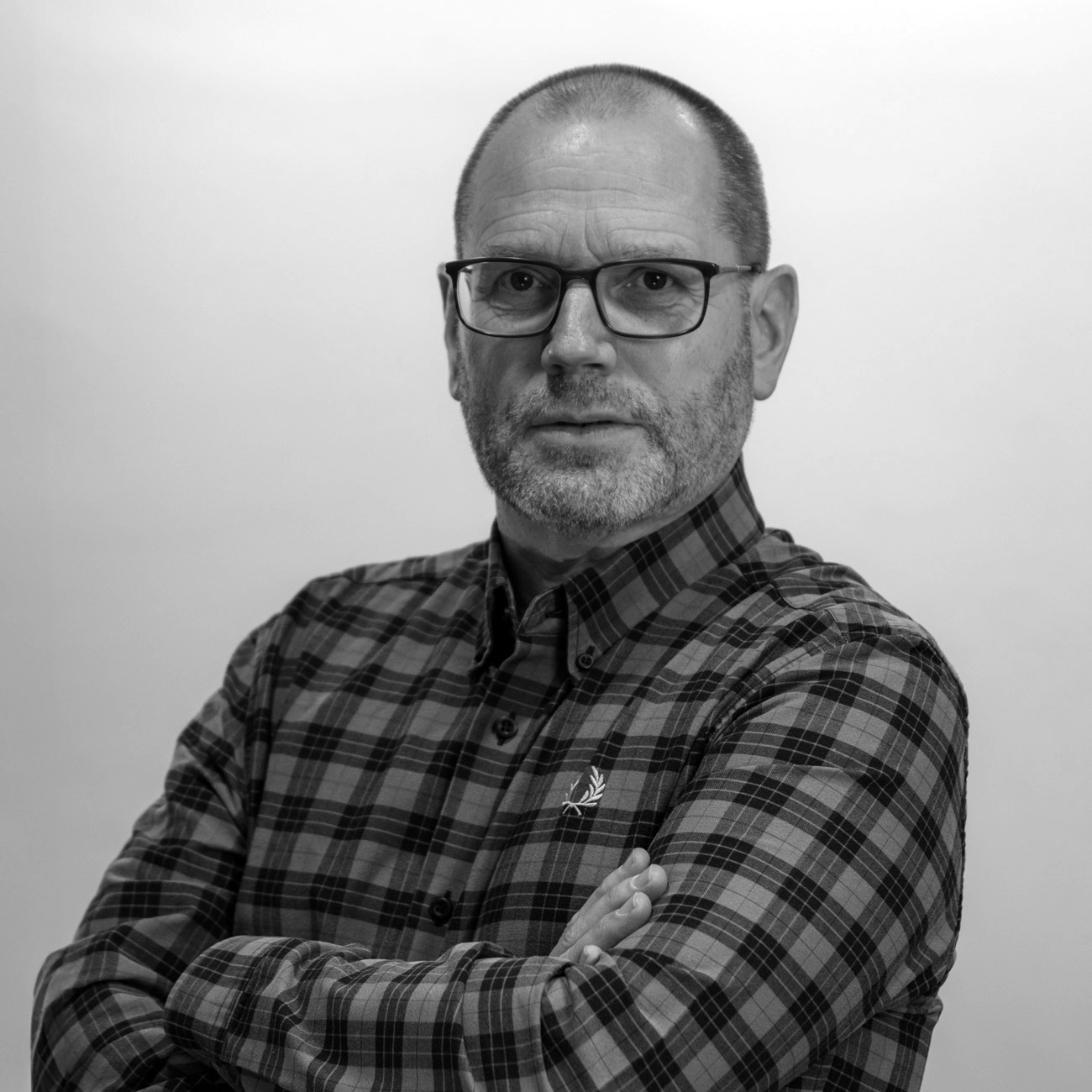
Published by David Sharp,
5 Jan 2024
The impact of AI is often described in terms of extremes
The impact of AI is often described in terms of extremes. On the one hand, it’s seen as a technological breakthrough to allow us to transcend the physical limits of our human bodies, which could let us live forever. On the other hand, the moment AI becomes intelligent enough to surpass us, it could be the end of humans and humanity altogether.
There are some big names in Camp Fear. Shortly before his death, Stephen Hawking wrote an open letter cautioning of the potential pitfalls if the risks of AI are not properly managed. Speaking in China two years ago, Elon Musk warned of AI as a “potential danger to the public”. Musk has described ‘cutting edge’ AI as being “far more dangerous than nukes”.
So, which is it? The end of the beginning, when AI helps to accelerate our ability to solve complex problems and frees us from a life of tyranny? Or the beginning of the end, when AI surpasses our ability to control it, and ends up subjecting us to a life of tyranny?
And how does the big picture play out in the field of health, safety and wellbeing? Let’s start with the opportunities.
Artificial intelligence is the name given to what is commonly known as machine learning – the ability for computers to teach themselves by using algorithms to process and analyse data and learn from the patterns they uncover.
AI is booming. An exponential growth in processing power, combined with the vast increase in the amount of data that’s now being collected, means that AI technologies can make existing processes much more efficient, powering entirely new ways of working that simply wouldn’t have been possible before. The European Agency for Safety and Health and Work divides these into three task-based solutions – information-related, person-related, and task-related.
Information-related tools include the growing use of process and document management software, such as the use of mobile phones to capture real-time photographic and video information that can feed into risk assessments and method statements, or workflow automation tools that design tasks to be carried out in the most efficient way possible.
You could add to this list the use of AI-driven image recognition cameras to monitor safe working practices such as compliant PPE use – things like checking that hard hats are being worn on a construction site – or to manage safe conditions such as ensuring that fall arrest equipment is anchored properly.
Looking at the interaction between information-related systems and people, there’s a growing use of wearables to keep track of workers and their equipment, either intrusively through requiring workers to wear wrist-based devices or carry swipe cards, or unobtrusively through tagging objects and equipment. Combined with geolocation data, AI can be used to support a whole range of safe-working processes, such as geofencing (using data to alert workers they are entering or leaving a specific safety zone) or customising data for the specific combination of worker, task and location in question. A system with this data processing capability will have no problem knowing that a team of maintenance engineers has arrived at a specific building to carry out planned repairs, and can issue a site briefing at the point of need. If one of the team tries to use a tool that they haven’t been trained on, the trigger can be automatically deactivated to prevent it.
Moving across further to the space where object- and people-related tasks overlap, AI starts to move from the mundane and barely visible, to the realms of science fiction. Virtual and augmented reality (VR and AR) – and the combination of them both through XR – can play a tremendously powerful role in health and safety training and communications. Using VR to train engineers to work on live electrical equipment, for example, is an obvious way to reduce risk. And overlaying visual data onto the headset of someone performing a complex task can both help them complete it safely, by acting as a real-time checklist, and learn from it by doing it.
What all these all-driven solutions have in common is that they undeniably bring benefits to the sphere of occupational health and safety. They reduce risk. They aid compliance. And they improve efficiency. So, what’s the problem?
It’s not that there’s a problem. Rather that these opportunities bring with them ethical challenges and pitfalls, which are too often overlooked. Let’s look at data and privacy concerns first.
The raw material that artificial intelligence feeds on is data. Because AI needs data to train its complex algorithms, the more data it can process, the more it can constantly learn and tweak the way it performs. Without large datasets, the so-called ‘knowledge’ that algorithms can gain – or the conclusions they can come to – can be very fragile. Remember that AI doesn’t actually understand the data it’s been given. It’s just looking for, and learning from, the patterns in that data. It doesn’t have what humans would call ‘common sense’ to understand when correlations between two sets of data are obviously spurious.
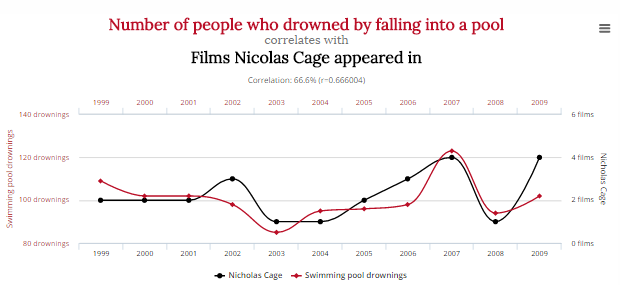
Shift in the interaction between humans and technology
Let’s look at an example. In this case, the correlation between the number of people in the US who drowned by falling into a pool, and the number of films that Hollywood actor Nicolas Cage appeared in. For the 11-year period from 1999 through to 2009, there is an uncanny correlation between the number of people who drowned falling into a pool, and the number of films Nicolas Cage appeared in. His appearance in Werewolf Women of the SS in 2007 coincided with the highest number of drownings during this time. As you can see from the graph, there is most definitely a correlation. The lines map really closely. But is there causation? Did those 120 people drown because Nicolas Cage made those movies? Did Nicolas Cage make those movies because that record number of people died?
A human would know that both suggestions are ridiculous and there is obviously no causal link between these two datasets. But an AI wouldn’t. It doesn’t know what pools are, or people are, or what films are, or who Nicolas Cage is. It would only know a causal link was highly unlikely if it had masses of additional data to put such a ridiculous conclusion into context. So it needs masses of data to do its job well.
We’ve all heard of the ‘Internet of Things’ – or IoT – where the sensor in our fridge talks to the app at the supermarket and automatically re-orders milk when it knows levels are low. All of this is supposedly for our benefit. To give us tools to make our lives easier. But what has actually happened in far too many cases is that the data being collected and correlated is not being done for our benefit – but rather for the benefit of the companies that process it, who sell it on for profit. The rules around data privacy are very slowly catching up, but regulators are still trailing in the wake of companies such as Google and Facebook who recognised the importance of our data and decided to ‘go for it’ some years ago – as Mark Zuckerberg did – when deciding what was acceptable to do with it.
What was previously considered as waste – known as exhaust data – had suddenly become valuable. Companies started to process it, link it to other data, sell it on, and make predictions based on it.
There has been a huge shift in the interaction between humans and technology, so subtle that we have barely noticed it. While we think of ourselves as being the beneficiaries of data – all these AI solutions designed to make our lives easier – what has actually happened is that we have become generators of data.
AI’s hunger for data shouldn’t be underestimated. Every last bit of data is being tracked – and even if it’s not being processed today, it can be stored for long periods of time or even indefinitely – frozen in time to feed into applications that haven’t even been built yet.
Last month saw the clearest evidence of this yet in the UK, when the Information Commissioner’s Office fined facial recognition company Clearview AI £7.5m for failing to follow data protection regulations. It’s the fourth time Clearview AI has been fined and ordered to delete data, following similar actions by governments in Australia, France and Italy.
Under the ruling, Clearview was found to have failed to meet the requirement to process data in a way that was fair and transparent, it failed to show a lawful reason for processing people’s data, and failed to have a process in place to stop data being retained forever. The company claims to have 20 billion images scraped from the public internet, including apps like Facebook and Instagram, and including images of UK residents. Though it no longer offers its services in the UK, it’s still selling those images – which might include your face or mine – to companies in other countries.
The more cameras, the more sensors, and the more compute power possible, the more data can be captured. Of course the argument goes: the more data, the more benefits AI can potentially bring to help us live and work safely and securely. You can imagine how a company like Clearview AI can present its data processing as beneficial. Issuing wearables to workers can help us protect them and help them work more efficiently.
Efficiency, control, safety and security are obviously important. But we shouldn’t forget the parallels between the present and the past. How does a worker issued with a wearable they are forced to wear, and which prevents them leaving certain areas, differ from the ankle bracelets issued by masters and worn by their slaves? Who is the master and who is the slave in the AI-human relationship?
One of the most volatile parts of the artificial intelligence landscape relates to what are known as large language models – huge algorithms that are trained on billions of bits of data scraped from across the internet. LLMs, as they are known, are really important. They’re the engines that drive voice assistants such as Siri and Alexa, and applications such as Android Auto, and some form of language model will power most AI applications.
The output from LLMs is highly dependent on their source data or corpus, as it’s known – in many cases populated from publicly available information on the internet such as Reddit boards or Wikipedia entries. Unfortunately, progress here – in the form of ever bigger models that capture more and more of the internet – is retrogressive: the more such AI models learn, the more likely they are to digest and repeat inaccurate information, and the less reliable they become.
So while LLMs can and do power automated conversational agents, their use in a mental health chatbot for workers might be not be advisable. This may sound obvious, but there has been an enormous growth in chatbots in recent years, with 325,000 of them created on one platform alone.
Whether it’s a piece of software such as a risk assessment or workflow distribution system – or a piece of embodied AI such as a cleaning service robot or a self-driving vehicle – we tend to be so impressed by its efficiency that we overlook the hidden costs – both financial and environmental.
How many times do you hear people saying proudly that they’ve bought an electric car because they want to be kind to the environment? Or that a company has switched its fleet? Yet we’re rapidly depleting some of earth’s most precious minerals. One car company – Tesla – is taking half the planet’s total resource of lithium hydroxide. Each Tesla Model S electric car needs just over 60kg of lithium for its battery pack, the weight of a small adult.
From 2012 to 2018, the amount of computing resource required to train the best-in-class AI model doubled on average every 3.4 months – a 3,000-fold increase in six years. Training is the important part of making sure an AI works properly. It takes time to feed in data, validate its performance, and test it. Training a single AI model was estimated to use as much energy as a trans-American flight, about a tonne of CO2. Each time you modify an AI, it has to be re-trained. Researchers recording the concurrent training of 4,789 versions of an AI model a couple of years ago noted the process took 172 days, a total compute time of 27 years, equivalent to 60 processors running every day for the six-month project.
Training an AI to work ‘autonomously’ is not cheap – financially or environmentally. And training an AI model takes considerably less power than operating one – the process known as inference. This is the bit where Alexa is working out what the next letter, word or sentence should be, based on every new piece of information it’s been given. Operating a large language model is reckoned to take about 80-90% of the total compute cost.
I’m an advocate of responsible AI; machine learning plays an important role in our own business, and I think it has a great deal to offer. But as a tool for use in occupational health and safety, my key takeaways would be:
-
Don’t assume that a technology solution is the best solution, just because it offers greater efficiency.
-
Remember that AI’s hunger for data is likely to see you collecting and processing vastly more data than is strictly needed – and that it is difficult to separate this out from the data you do need. Employing AI solutions can be like using a sledgehammer to crack a nut.
-
Artificial intelligence is narrow and brittle. It does not have common sense, and its outputs cannot necessarily be trusted.
-
Running AI solutions – both in terms of hardware and software – is not magic. AI as a process of extraction has an enormous but often unseen cost – on us as people, and on the environment.
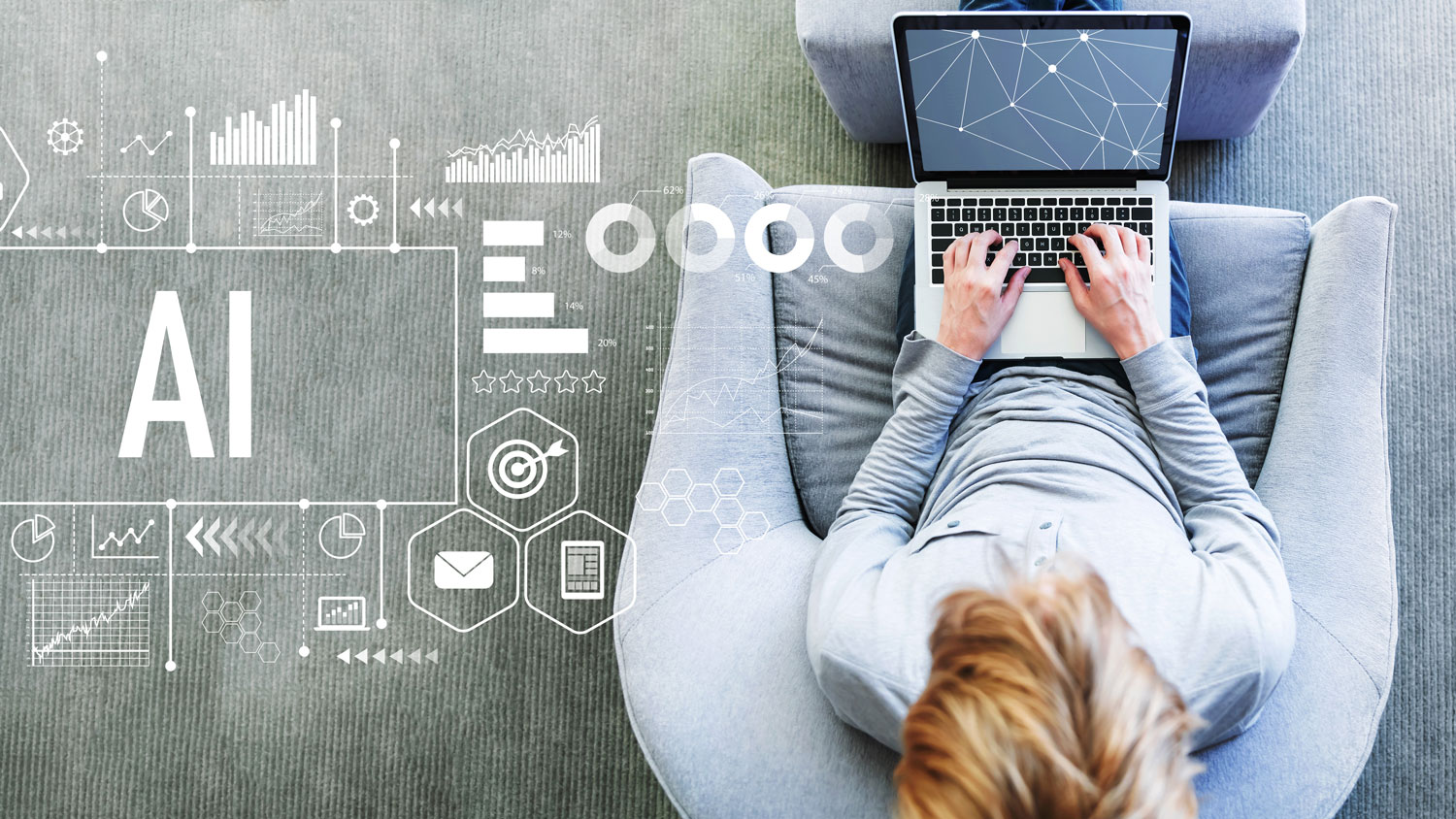
SOURCES:
- Crawford, K. (2021) Atlas of AI: Power, Politics, and the Planetary Costs of Artificial Intelligence. New Haven: Yale University Press.
- Pearl, J. and Mackenzie, D. (2018) The Book of Why: The New Science of Cause and Effect. London: Penguin Books.
- Véliz, C. (2020) Privacy is Power: Why and How You should Take Back Control of Your Data. London: Transworld Digital.
- Zuboff, S. (2019) The Age of Surveillance Capitalism: The Fight for a Human Future at the New Frontier of Power. First edition. New York: PublicAffairs.